AI-powered lead qualification saves businesses time by working around the clock to find the best potential customers. It pulls up-to-date data from many parts of a website, ensuring fresh and accurate information is used to evaluate leads. This approach makes it easier to focus on high-quality leads that are most likely to become loyal customers.
This technology analyzes data quickly and automates much of the sales process, making it faster to pinpoint the perfect potential customers. AI-powered lead qualification organizes and prioritizes leads, helping sales teams respond more efficiently. Because it can operate 24/7, businesses can move through their sales pipeline faster, reducing delays and improving results.
With AI-powered tools handling lead qualification, sales teams can focus on building relationships and closing deals. This not only saves time but also boosts productivity and revenue. Businesses using AI for sales automation often see significant improvements in customer targeting and overall performance. Let AI handle the heavy lifting so your team can focus on what they do best—selling.
Key Takeaways
- AI-powered lead qualification integrates data from various sources, enabling faster identification of high-value prospects.
- Machine learning algorithms and predictive analytics improve accuracy and speed in lead scoring and qualification.
- AI enables personalized lead nurturing based on behavior and preferences, enhancing engagement and conversion rates.
- Real-time data integration and dynamic lead-scoring models are key features of AI tools aimed at quick prospect identification.
- Future trends in AI and sales include further automation and hyper-personalization, boosting the efficiency of pinpointing the best prospects.
Understanding AI-Powered Lead Qualification
In sales and marketing, AI-powered lead Qualification has emerged as a game-changing tool. This technology integrates data from a myriad of sources to construct thorough lead profiles. The data amalgamation from website interactions, CRM systems, and social media feeds into machine learning algorithms, such as predictive analytics, clustering, and natural language processing.
These algorithms underpin the AI-powered lead scoring models, which assign numerical values to leads based on demographic fit, behavioral engagement, and company data. Continual refinement of these scores guarantees attention is directed towards the most promising leads. The AI technology’s capability to automatically analyze lead data, recognize patterns, and identify high-value leads undeniably provides a competitive edge.
Key components of this process include defining an Ideal Customer Profile (ICP) through historical data analysis and gathering extensive lead data. The AI system then segments leads, excluding unqualified ones and prioritizing high-value prospects. Instantaneous processing of lead data and predictive analytics further improve the accuracy and efficiency of lead qualification. This clear understanding of AI-Powered Lead Qualification opens the door to freedom in sales and marketing strategy. This technology also integrates seamlessly with existing sales tech stacks, ensuring efficient data flow and better decision-making (efficient data flow). In addition, this technology enables businesses to leverage automated tools to predict customer behavior, thus driving an increase in sales revenue.
Benefits of AI in Lead Qualification
Utilizing the power of artificial intelligence in lead qualification delivers an abundance of substantial benefits. Improved efficiency is an immediate advantage of AI integration, as it automates lead routing and notably reduces qualification time. AI’s ability to streamline data analysis and improve response times, coupled with its capability to operate continuously, guarantees no potential leads are overlooked.
Another key benefit is the accuracy afforded by AI. AI employs predictive analytics, machine learning, and natural language processing to score leads based on their likelihood of becoming customers. Furthermore, it offers an extensive view of a lead’s engagement, adjusting to new patterns and updating scores based on the latest information. This precise data-driven decision-making is a key feature of sales automation that AI brings to the table.
AI excels at processing leads in real-time. It analyzes thousands of data points instantaneously, continually updating scores as new information surfaces. Moreover, AI automates the initial screening process and operates around the clock, leading to shorter sales cycles.
Finally, AI enables individualized nurturing of leads. It personalizes messages and segments leads dynamically based on behavior and preferences, improving the overall customer experience. Essentially, AI-powered lead qualification is a powerful tool for pinpointing your best prospects faster. Significantly, by focusing on high-quality leads, companies can drive revenue growth, making AI an instrumental tool in achieving business goals (revenue growth).
Implementing AI for Lead Qualification
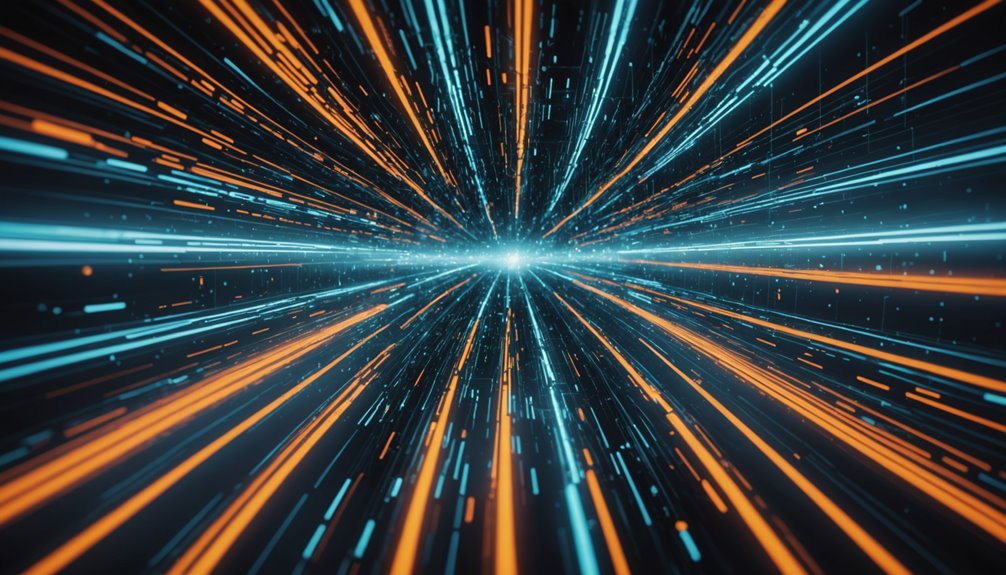
Implementing AI for lead qualification necessitates a tactical method, beginning with the selection of the right AI solution that aligns with your business requirements and integrates seamlessly with existing systems. Optimization is a continuous process, requiring regular adjustments to AI models based on emerging data and insights to improve accuracy and efficiency. AI tools such as predictive analytics can drastically improve the lead qualification process by forecasting consumer behaviors and trends, increasing the efficiency of identifying potential clients. The integration of AI can effectively transform the lead qualification process, enhancing both the assessment and engagement dimensions. Hence, a combination of careful AI solution selection, consistent model optimization, and strategic AI integration can markedly improve the effectiveness of lead qualification processes.
Choosing the Right AI Solution
Selecting the appropriate AI solution for lead qualification necessitates a meticulous assessment of several essential factors. Data integration capabilities are critical, guaranteeing the solution can merge information from diverse sources like CRM systems, marketing automation platforms, and social media. The selected AI solution must demonstrate algorithmic complexity, equipping it with predictive capabilities to score leads accurately.
Another vital criterion is customization, which allows Bayou to tailor lead qualification parameters to your specific business needs. Scalability guarantees that the system can manage large data volumes and expand your business. Security and privacy measures are vital to assure trustworthiness and compliance.
Key features to seek include automated lead scoring, predictive insights, lead profiling, and continuous processing. Smooth integration with existing systems is also essential, enhancing usability and data consistency. This will help in streamlining processes and workflows(#), ultimately improving efficiency and productivity.
However, potential implementation challenges such as high initial costs, complexity, resistance to change, data quality issues, and training requirements need careful consideration. Additionally, it’s essential to have access to comprehensive support resources, which can help users navigate the system effectively and troubleshoot any issues. Extensive support resources can make the difference between a successful and unsuccessful AI implementation.
Ultimately, defining clear criteria for lead qualification, thoroughly evaluating AI providers, validating data accuracy, and committing to continuous optimization are best practices for successfully implementing AI for lead qualification.
Optimizing AI Models Regularly
Regular optimization of AI models is critical to successful lead qualification. This process involves automated data import, continuous model training, and predictive analytics that adapt to changing market dynamics and customer behaviors. It enables businesses to maintain the accuracy of their AI models and improve lead qualification with advanced algorithms that identify patterns and correlations indicative of a lead’s quality and readiness to buy.
Real-time processing and regular updates improve accuracy and efficiency. By analyzing large datasets in moments, AI models can quickly qualify leads and prioritize follow-ups, eliminating bottlenecks. As these models refine themselves over time, they provide more tailored messaging suited to distinct leads, enhancing conversion likelihood.
Moreover, the integration of generative AI in lead qualification has been a game-changer, offering unparalleled insights and predictions about potential customers based on a wide array of factors such as demographics, online behavior, and past interactions (a wide variety of factors). This advanced system also allows for the segmentation of leads, increasing engagement and lead conversion.
However, the process isn’t without challenges. Initial costs can be high, and resistance to change, complex learning processes, and data integration can pose difficulties. Despite these challenges, regular optimization, data validation, continuous monitoring, and integration with CRM systems are best practices, ensuring reliable lead qualification and seamless conversion from marketing-qualified leads (MQLs) to sales-qualified leads (SQLs).
Strategies for Effective AI Lead Qualification
How can businesses effectively utilize the power of AI for lead qualification? The key is to adopt a tactical method that combines technology and data-driven insights.
Firstly, businesses need to define clear qualification criteria that align with their Ideal Customer Profile. These attributes, which may include demographic, firmographic, and behavioral factors, will guide the AI’s analysis.
Next, choose a lead qualification solution that integrates seamlessly with your existing platforms. These systems should be capable of combining data from multiple sources, including website interactions, CRM systems, and social media, and applying machine learning algorithms to identify patterns and score leads.
Continuous optimization of AI models is also essential. Regularly feeding new data into the system guarantees its accuracy and ability to adapt to changing customer behaviors. Moreover, successful AI lead qualification has proven to transform anonymous visitors into qualified prospects, significantly improving conversion rates.
The integration of AI with CRM systems is paramount in streamlining the lead qualification process, making interaction tracking more efficient, and improving team communication.
Businesses should also measure the ROI of their AI lead qualification efforts. This will help them assess their effectiveness and make necessary adjustments.
Finally, prioritize lead scoring. Use AI to assign numerical values to leads based on their predicted likelihood of conversion. This will help sales teams focus their efforts on the most promising opportunities.
Following these strategies will help businesses capitalize on the power of AI to qualify leads more effectively and efficiently.
Key Features of AI-Powered Tools
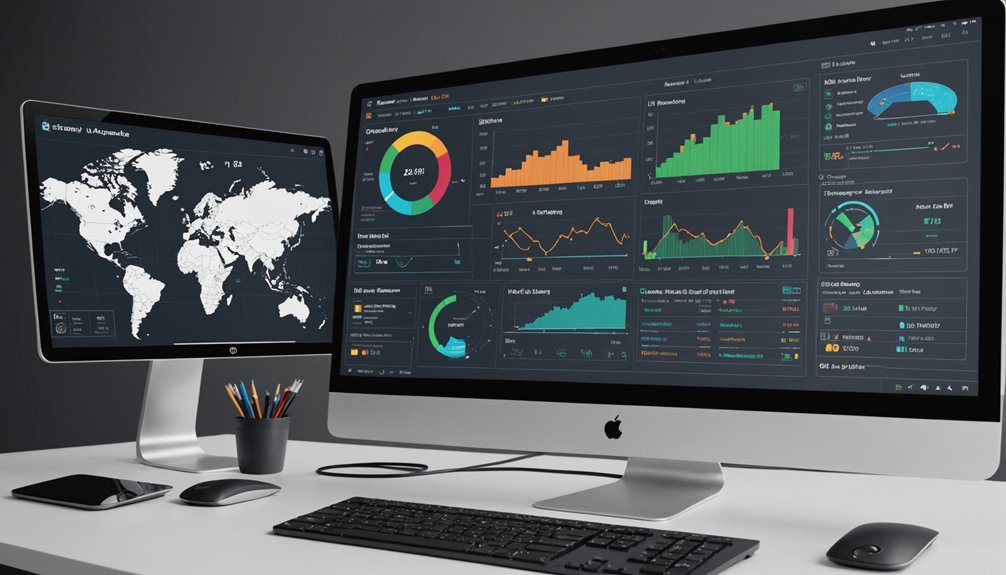
What distinguishes AI-powered lead qualification tools from traditional methods? The answer lies in their advanced, data-driven features that transform lead qualification processes. One key feature is the ability to integrate and analyze data from multiple sources in actual time, including historical data and behavioral interactions. This thorough data analysis allows AI to build robust lead profiles and make accurate predictions about lead behavior and conversion likelihood.
AI-driven lead scoring is another groundbreaking feature, offering dynamic and tailored scoring models based on demographic fit, behavioral engagement, and company data. Instant prioritization and automated lead routing guarantee that sales teams always focus on the most promising leads.
In addition to these features, AI-powered tools can also effectively handle missed calls, providing a solution for a common issue that can have significant financial implications, including a potential revenue loss of $20 per call.
AI-powered tools also improve lead qualification processes through automated monitoring, individualized nurturing, efficient handoffs, and enhanced accuracy. By leveraging large datasets and minimizing personal error, AI dramatically reduces the time required to qualify leads.
Advanced AI capabilities, such as natural language processing, multichannel data integration, predictive insights, and continuous optimization, further enhance these tools’ functionalities. With AI, sales teams can enjoy data-driven segmentation, allowing for targeted engagement that meets specific lead profiles and needs. Moreover, the ethical handling of customer data is a crucial consideration, as AI tools must adhere to strict privacy regulations to maintain trust (ethical practices).
Real-World Applications and Examples
AI-powered lead qualification has already made a significant impact in the business landscape, as seen in the success narrative of a B2B company that experienced a 30% increase in its sales pipeline. By using AI, businesses can more efficiently allocate resources, qualifying leads up to 50% faster than traditional methods. Furthermore, AI’s ability to process fresh data and update lead scores in real time offers a scalable solution for lead qualification, highlighting the practicality of its practical applications.
B2B Company Success Story
Drawing from the domain of tangible achievement narratives, we find compelling examples of B2B companies that have utilized the power of AI for lead qualification. Acme Solutions, a B2B software company, implemented AI-powered lead qualification, which resulted in a 30% increase in its sales pipeline and a 15% improvement in conversion rates. This was achieved by using AI models to analyze vital lead data, leveraging automation and scalability to handle vast amounts of data.
Another achievement narrative comes from Global Tech Inc., a multinational technology company that utilized AI for lead scoring. This led to a 40% reduction in lead qualification time and a 20% increase in sales win rate. Here, the AI system integrates data from multiple sources to build thorough lead profiles and uses predictive analytics to identify patterns indicating a lead’s quality.
The case of Velocity Marketing further demonstrates the revolutionary power of AI. The marketing agency automated its initial lead qualification process using AI, reducing its lead qualification time by 60%. This was achieved through AI’s ability to learn from historical data and continuously refine its models, ensuring the accurate evaluation and prioritization of leads.
Efficient Resource Allocation
Efficient resource allocation, particularly in lead qualification, has become a strategic advantage for businesses that utilize artificial intelligence. AI’s potential to improve efficiency is realized through faster lead identification, reduced manual effort, improved accuracy, immediate processing, and the elimination of bottlenecks.
From a practical perspective, the key components of an AI-powered lead qualification system include data integration, machine learning algorithms, lead scoring models, continuous optimization, and predictive analytics. These components work synergistically to deliver a streamlined, efficient, and effective lead qualification process.
To illustrate, consider the following practical applications:
- Companies like Acme Solutions have witnessed a 30% increase in their sales pipeline and a 15% improvement in conversion rates by leveraging AI-powered lead qualification tools.
- AI has markedly reduced the time required to find and qualify leads, enabling faster response times and improved sales efficiency.
- The collaborative potential between marketing and sales teams has been improved, ensuring a cohesive and precise approach to identifying and pursuing quality leads.
Such results underscore AI’s groundbreaking potential. AI enables businesses to allocate resources more efficiently and effectively in their lead qualification processes.
Scalable Lead Qualification
Building upon the foundation of efficient resource allocation, it’s crucial to investigate the scalability of lead qualification, mainly as it manifests in authentic-world applications and examples. AI-powered lead qualification systems provide a scalable solution, enabling businesses to manage vast amounts of data and quickly identify high-quality leads.
For instance, B2B companies are leveraging AI to improve their sales pipelines, accurately predicting conversion likelihood and increasing revenue growth. Moreover, these systems can adapt to changes in data patterns, refining their models over time for improved precision. This scalability is evident in actual-world examples, with businesses reporting significant improvements in conversion rates.
Consider the following table for a snapshot of actual-world applications and their outcomes:
Company | Implementation | Outcome |
---|---|---|
Company A | AI-based lead scoring | 30% increase in conversions |
Company B | Machine learning for data analysis | 20% growth in revenue |
Company C | Real-time data processing | Reduced lead qualification time |
Company D | extensive lead profiling | Improved sales pipeline efficiency |
Company E | tailored nurturing strategy | Improved customer relationships |
Scalable lead qualification powered by AI is a game-changer, offering the potential for rapid growth.
Overcoming Challenges in AI Adoption
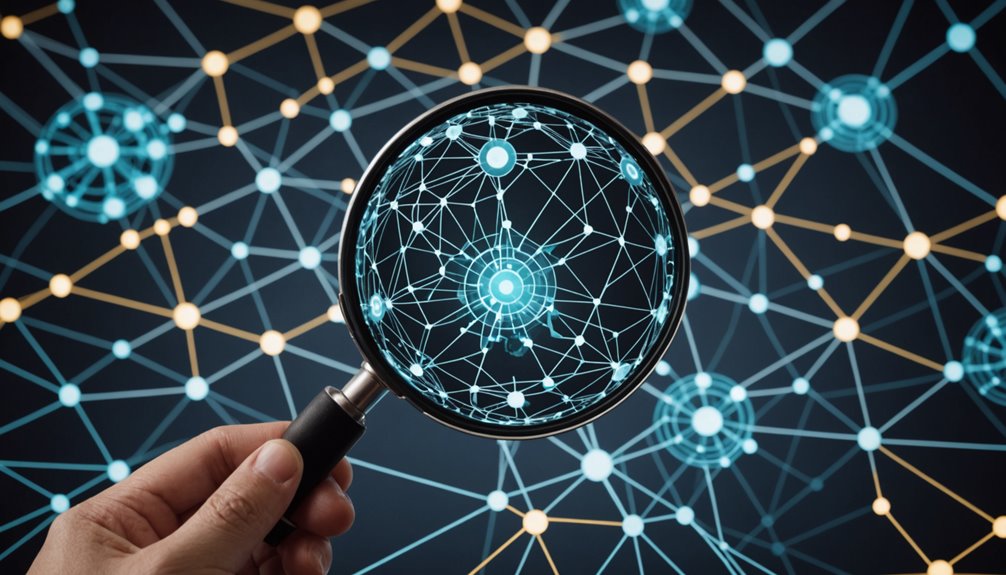
Maneuvering the complex maze of AI adoption presents a myriad of challenges, ranging from data quality issues to talent shortages. A strategic vision, coupled with a deep analysis of business processes, helps identify areas where AI can have the most impact. However, the path to successful AI adoption is also riddled with technical challenges and cybersecurity risks, necessitating robust defense strategies and continuous improvement of AI systems.
To help navigate these challenges, consider the following strategies:
- Pilot Projects: Initiating pilot projects allows for testing and refinement of AI models before full-scale implementation. This mitigates risks and enables organizations to understand AI capabilities better.
- Cross-Functional Teams: Engaging cross-functional teams in AI implementation fosters a collaborative environment, bringing together varied expertise to address challenges and find the best use cases for AI.
- Continuous Monitoring and Adjustment: Regularly reviewing and adjusting AI algorithms based on performance data and market trends guarantees the technology remains adequate, relevant, and optimized for lead qualification.
Future Perspectives: AI and Lead Qualification
As we gaze into the future of lead qualification, the advent of AI-powered solutions is impossible to ignore. Advanced machine learning algorithms and predictive analytics will transform the process by analyzing vast data sets to identify patterns, anticipate prospect behavior, and provide accurate lead prioritization. This data-driven approach also enables hyper-personalization, tailoring interactions to distinct prospect needs for improved engagement.
Advancements in AI technology, such as natural language processing and deep learning, are set to define this process and improve communication efficacy and data analysis. Moreover, integrating data from multiple channels provides a holistic view of lead behavior, leading to improved qualifications.
Sales teams can expect increased efficiency and improved conversion rates. AI automation of lead qualification will allow sales professionals to focus on high-potential leads, and the provision of practical insights will amplify decision-making abilities.
Future trends suggest additional automation and improved hyper-personalization, with AI integrating with emerging technologies for a more nuanced approach. In this future landscape, sales teams will be equipped to engage leads more effectively, boosting the potential for successful conversions.
Frequently Asked Questions
What Are the Costs Associated With Implementing AI for Lead Qualification?
The costs of implementing AI for lead qualification encompass development, training, infrastructure, and maintenance expenditures. They also include the potential investment in AI models, data preparation, and personnel such as data scientists and engineers.
How Secure Is the Data Used in Ai-Powered Lead Qualification Systems?
Data in AI-powered lead qualification systems is secured through encryption, data privacy compliance, and regular audits. Data validation and continuous monitoring also guarantee accuracy and prevent breaches, safeguarding the quality of lead predictions.
What Challenges Might a Company Face When Transitioning to AI for Lead Qualification?
Shifting to AI for lead qualification presents challenges, including data quality issues, integration complexities, the need for training, the risk of overreliance, cost considerations, lack of transparency, and potential bias in AI models.
Can Ai-Powered Lead Qualification Tools Integrate With All CRM Systems?
While most AI-powered lead qualification tools are designed to be compatible with popular CRM systems, full integration may depend on the specific CRM system and tool. Tailorable integration options can improve compatibility across diverse systems.
How Does Ai-Powered Lead Qualification Affect the Role of Sales Representatives?
AI-powered lead qualification transforms sales representatives’ roles by automating routine tasks, enhancing lead targeting accuracy, and providing practical insights. This enables them to focus more on nurturing high-potential leads and closing deals efficiently.
Conclusion
To summarize, integrating AI in lead qualification has the potential to transform sales and marketing strategies. By fine-tuning prospects faster and more precisely, organizations can optimize their resources and increase general efficiency. However, the full realization of these benefits hinges on overcoming challenges in AI adoption and continuously refining AI-powered tools. As AI continues to evolve, so too will its impact on lead qualification, promising a future of improved business performance.
0 Comments